Review of The Language of Thought: A New Philosophical Direction by Susan Schneider
2019 Mind, 128: 555–564
Last updated 17 May 2019
Contents
- 1 Introduction
- 2 Central reasoning is not computational
- 3 What is a LOT symbol?
- 4 Concepts and Frege cases
- 5 Conclusion
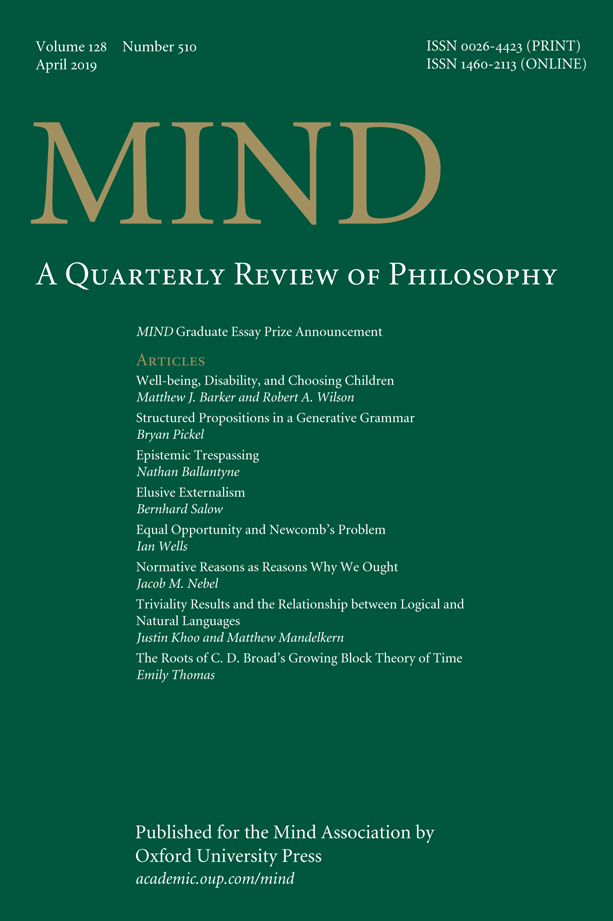
1 Introduction
The Language of Thought (LOT) is closely associated with the work of Jerry Fodor. He defended the idea in his book, The Language of Thought (1975), and continued to do so, with relatively minor revisions, throughout his career. Susan Schneider’s book does not aim to be an exegesis or defence of Fodor. Instead, it offers an alternative to Fodor’s version of LOT that she says is an improvement and a worthy replacement. Her aim is to overcome three challenges that faced his approach.
According to both Fodor and Schneider, LOT’s goal is to explain human thought in naturalistic, mechanical terms. Schneider defines LOT as a package of three claims to this end. First, having a thought involves tokening ‘symbols’ in your head and combining those symbols into well-formed symbolic expressions according to language-like grammatical and semantic rules. Second, thinking is a computational process involving LOT symbols and symbolic expressions. Third, the semantic value of an LOT symbol is determined by it standing in a naturalistic causal or nomic ‘locking’ relation to entities in the world.
Schneider says that Fodor’s version of LOT faces three challenges:
- Central reasoning is not a computational process
- The notion of a LOT symbol is unclear
- LOT is unable to handle Frege cases
In this review, I will describe the three challenges and Schneider’s proposed solution. As will become clear, I don’t entirely agree with everything Schneider says. Especially with respect to her answer to (2), I think that her version of LOT incurs costs that should lead us to question it. But notwithstanding this, my overall impression of her book is a positive one. This book will undoubtedly set the agenda for future work on LOT. It places the often-ignored problem of the nature of LOT symbols at the centre of the LOT debate and it shows how solutions to this problem reach out and touch many other aspects of the theory. The quality of scholarship and writing is high throughout. Unusually for a philosophy monograph, it is also fun to read.
2 Central reasoning is not computational
Fodor famously argued against LOT as a theory of central reasoning. Fodor defined central reasoning as non-demonstrative reasoning that is sensitive to all (or nearly all) of one’s beliefs. Central reasoning is meant to cover processes such as how we revise our beliefs in light of evidence, how we make inductive inferences, and how we construct practical plans to achieve our goals. According to Fodor, two problems stop LOT from being able to account for central reasoning: the globality problem and the relevance problem. (These are sometimes misleadingly called the ‘frame problem’; see Shanahan (1997) for a description of the real frame problem.)
First, the globality problem. Fodor said that certain properties of individual representations – their simplicity, centrality, and conservativeness – are ‘global’ in the sense that these properties vary with context; they are not intrinsic to the representations of which they are predicated. Sometimes adding a certain belief to one’s belief set will complicate a plan; sometimes it will simplify it. A belief’s ‘simplicity’ does not supervene on that belief’s intrinsic properties. Therefore, it does not supervene on a belief’s syntactic properties. Computational processes are sensitive only to syntactic properties. So, says Fodor, reasoning that requires sensitivity to global properties cannot be a computational process, and thus falls outside the remit of LOT.
Schneider, in a chapter co-written with Kirk Ludwig, responds that a computer is not sensitive merely to the syntax of individual representations. A computer is also sensitive to syntactic relations between representations: how a representation’s syntax relates to the syntax of other representations and how these relate to the system’s general rules of syntactic processing. The failure of an individual representation’s simplicity to supervene on the representation’s syntactic properties does not mean that simplicity cannot be tracked by a computational process. Simplicity may supervene on (and be computationally tracked by following) syntactic interactions between representations. It is worth noting that Fodor (2000) considered this possibility too in a view he labels M(CTM). However, he argued that this solution would run into the relevance problem, shifting attention to the other part of his argument. (See Samuels (2010) for a helpful reconstruction of Fodor’s argument here.)
The relevance problem arises because central reasoning has access to a large number of representations: potentially all of the system’s beliefs, desires, and thoughts. Any one of these could be relevant to the system’s reasoning in any given case, but usually only a few are. The human central reasoning system tends to focus on just those representations that are relevant to the agent’s current goals, plans, and context. How does it know which representations are relevant without doing an exhaustive, impracticable search through its entire database? Fodor says we do not know of any computational process that would solve this problem. (We don’t know of any non-computational process either, but never mind that.) He says that the difficulty of the relevance problem explains our failure to produce a computer with artificial general intelligence (AGI). Successful AI systems tend to excel at narrowly defined tasks (like playing Go or detecting your face), but they do not show general intelligence: they are poor at pulling together relevant information from disparate sources to make plans outside their narrowly defined area of competence.
Building on work by Shanahan & Baars (2005) and Dehaene & Changeux (2004), Schneider argues that a solution to the relevance problem can be found within Global Workspace Theory (GWT). GWT says that multiple ‘specialist’ cognitive processes compete for access to a global cognitive ‘workspace’. If granted access, the information a specialist has to offer is ‘broadcast’ back to other specialists. Access to the global workspace is controlled by ‘attention-like’ processes. The contents of the global workspace unfold in a largely serial manner over time. Schneider identifies the serial unfolding with central reasoning, and she argues that the relevance problem is solved by the ceaseless parallel work of the specialists.
I am not convinced by this solution. GWT describes a functional architecture – and in the case of its neuronal version, an anatomical architecture – that the brain could use to share and manage information. In this respect, GWT pertains to part of the relevance problem: in order to bring information to bear in central reasoning there must be channels to share and manage information. But, and it is an important but, GWT does not say how traffic along those channels is regulated to guarantee relevance. It does not explain how relevant, and only relevant, information is shepherded into the global workspace. Shanahan and Baars don’t attempt to explain this, and neither does the more neurally orientated GWT work. The answer cannot be bottom-up pressure from specialists (for there is no reason to think that the specialist who shouts loudest contains relevant information); it also cannot be top-down selection by some executive process (for that would introduce the relevance problem for that executive process). How then does the reasoning system ensure that relevant, and only relevant, information filters into the global workspace? If the answer is ‘attention’, what mechanism keeps attention aligned to what is relevant to the system in the current context? Baars & Franklin (2003) describe interplay between ‘executive functions’, ‘specialist networks’, and ‘attention codelets’ that control access to the global workspace. Unfortunately, how these components work to track relevance is left largely unspecified. A computational solution to the relevance problem may be compatible with GWT; but GWT, as it currently stands, is largely silent about how relevance is computed.
What would it take to find a computational solution to the relevance problem? Fodor linked this to our ability to build a working AGI. Schneider disagrees: she says this sets the bar too high. I do not think so. Building a working computational model that can engage in non-trivial non-demonstrative reasoning shows that we know how to solve the relevance problem; that we really know how to solve it and not just offload the hard parts onto an unexplained part of the model (for example, ‘executive function’, ‘attention’). Building an artificial simulation capable of solving the relevance problem is the hallmark that a computational solution to the problem has been found.
Fodor thought that we would never find a solution and he cited a long history of AGI failures in support. However, past failure is only a guide to the future if the computational techniques explored so far are representative of those that we will discover in the future. Fodor’s confidence in this strikes me as unfounded. Schneider may overreach when she says that GWT already solves the relevance problem, but her overall strategy – promoting the opportunities offered by novel computational architectures – strikes me as fundamentally correct. There are more computational architectures than were dreamt of in Fodor’s philosophy (or than we can dream of today). GWT is one, but there are many others. Deep Q-networks, completely unrelated to GWT, show promising elements of domain-general reasoning. A single deep Q-network can play forty-nine Atari computer games, often at super-human levels, switching strategy depending on the game it plays (Mnih et al. 2015). Significantly, the network is never told which game it is playing. It works this out for itself from the pattern of pixels it ‘sees’. The network pulls together, by itself, a policy relevant for playing the game in hand. This isn’t AGI or a solution to the relevance problem, but it’s a step in the right direction.
3 What is a LOT symbol?
LOT explains thought and thinking in terms of LOT symbols, but what is a LOT symbol? If you look inside someone’s head, you don’t see anything that looks like a symbol. How then should we understand LOT’s talk of symbols inside the head? Schneider calls this question the ‘elephant in the room’ for LOT. Fodor did little to address it; he focused instead on arguing for explanatory and predictive gains that would flow to psychology from positing LOT symbols, whatever those symbols happen to be.
If one is puzzled about what some thing is, a plausible opening gambit is to substitute the question of what it is with a question about its individuation conditions. This is Schneider’s strategy. Her question, then, becomes: when are two physical tokens – in particular, two brain states – of the same LOT symbol type?
Schneider discards two theories of LOT symbol individuation before proposing her own.
The first theory she discards is a ‘semantic’ theory. A semantic theory of symbols says that two physical tokens are of the same symbol type just in case they have the same semantic content. Schneider’s objection is that a semantic theory would conflict with LOT’s ambition to give a reductive, naturalistic theory of semantic content. LOT is committed to explaining the semantic content of LOT symbols in terms of naturalistic (causal or informational relations) relations between LOT symbols and the world. This reductive project won’t work if one of the players in the reductive base – LOT symbols – themselves depend on semantic content.
The second theory Schneider rejects is an ‘orthographic’ theory. An orthographic theory says that two physical tokens are of the same symbol type just in case they have the same ‘shape’. The ink marks on this page can be grouped into symbol types based on their physical shape. Obviously, ‘shape’ means something different for LOT symbols than it does for ink marks – you don’t find neurons shaped like the letter ‘a’. Schneider rejects the orthographic theory because it does not provide an account of this alternative notion of ‘shape’.
Schneider’s preferred theory individuates symbols by their computational role. Her theory says that two physical tokens are of the same symbol type just in case they play the same computational role within the computing system. Schneider defines ‘playing the same computational role’ as being physically interchangeable without affecting the computation. Two physical tokens play the same computational role just in case one physical token can be exchanged for the other without affecting any (actual or possible) computational transitions of the system. A key source of support for her view comes from John Haugeland’s account of formal symbol systems like chess:
Formal tokens are freely interchangeable if and only if they are the same type. Thus it doesn’t make any difference which white pawn goes on which white-pawn square; but switching a pawn with a rook or a white pawn with a black one could make a lot of difference. (Haugeland 1985 p. 52)
Furthermore, Schneider argues that physical tokens should be typed by their total computational role. That means that any change, no matter how small, to a system’s (actual or possible) computational transitions resulting from exchanging two of its physical tokens entails that those tokens are not of the same symbol type.
I will not describe the arguments that Schneider gives to support her theory. Instead, I wish to flag two potential problems.
The first is that her theory (and Haugeland’s) does not appear to work for more complex computers such as modern electronic PCs. Inside a PC, physical tokens of the same symbol type vary enormously in their physical nature; they are rarely freely interchangeable. Conversely, physical tokens of different symbol types can sometimes be interchanged without affecting the computation at all. This is because modern PCs, unlike chess sets, keep track of changes in their physical tokens and adjust their principles of physical processing accordingly. This strategy is called ‘virtualising’ the physical hardware. It occurs across multiple levels inside a PC (see Patterson & Hennessy 2011, Ch. 5). For example, suppose that a physical token of the symbol type ‘dog’ is tokened inside my PC (maybe as part of an email message). Imagine that this physical token involves electrical activity in my PC’s physical RAM locations 132, 2342, and 4562. However, these locations, and this pattern of activity, are not somehow reserved for ‘dog’ tokens. Nanoseconds later, tokening ‘dog’ may involve electrical activity in different physical RAM locations, say, 32, 42, and 234. Now, tokening ‘cat’ may involve electrical activity in the old physical RAM locations of 132, 2342, and 4562. The physical memory inside my computer is constantly being ‘remapped’ to optimise my computer’s performance. In such a context, using interchangeablity of physical tokens within the computation to individuate symbol types is hopeless. Tokens that play the same total computational role may not be freely physically interchangeable (‘dog’ now and ‘dog’ after a memory remap), and tokens that are freely interchangeable without affecting the computation may play different computational roles (‘dog’ now and ‘cat’ after a memory remap).
What happens inside a modern PC is that physical tokens that fall under the same symbol type vary but the PC’s physical principles of manipulation vary accordingly to accommodate the change. The PC’s formal principles for manipulating symbol types (its algorithm) stays constant throughout. (Below, I consider cases in which the algorithm changes too.) Imagine that, during a chess match, the physical board were cut up into pieces and reorganised after every move but the physical principles governing movement of the chess pieces were changed to accommodate the reorganisation: black’s king’s rook can now move to different squares and it is symbolised by a horse-shaped figure, but it can attack, and be attacked by, the same white pieces – the overall state of play in the game is unaffected. Only a lunatic would reorganise their chess board like this during a chess match. But physical remapping is both adaptive and common in electronic PCs. One might expect brains to use similar virtualising tactics given their benefits for squeezing optimal performance from limited computing hardware.
In summary, the first problem is that ‘same total computational role’ does not mean ‘physical interchangeability’, at least for computers that use virtualising strategies. The second problem is that Schneider’s account does not provide stable symbol types. She foreshadows this worry when she says that her proposal makes it hard for symbol types to be shared across different computers. You and I are not disposed to undergo exactly the same computational transitions when thinking about dogs, so we do not have the same LOT symbol types. (Maybe you have DOG1 and I have DOG2.) In a footnote on page 130, Schneider says that similar worries apply to a single human being over time. She has in mind relatively slow changes in someone’s computational roles that might occur over a lifetime. However, the difficulty comes not from slow changes, but from short-term changes produced by learning.
The algorithms run by electronic PCs are normally fixed, either by their hardware or by the program they are given. But computers can also modify their algorithms. Machine learning is now common. Computers like AlphaGo modify their (hugely complicated) algorithms in many ways in response to learning data (either labelled examples of ‘good’ behaviour or reward/punishment signals). When learning occurs, a computer modifies its algorithm: total computational roles before and after learning are different. This creates a problem for Schneider’s account of symbol identity. She indexes symbol identity to a symbol’s total computational role, but this role changes during learning. A change, even a small one, to a symbol’s computational role will ramify. Remember that any change to a computational role, no matter how minor, changes a symbol’s identity. Remember too that the computational role of a symbol includes not just the symbol’s actual computational transitions but also any possible transitions that it could undergo. A change induced by learning, even one that does not affect the actual processing of the symbol, is almost certain to affect some possible computational transition that the symbol could enter into – perhaps by affecting the computational roles of other symbols to which the symbol is related by a sequence of merely possible computational transitions. Unless the computational system is designed so as to minimise all computational relations between its symbols (and what would be the point of a machine like that?), small changes to a computational role will percolate throughout the system, changing symbol identities in their wake. The upshot is that Schneider’s symbol types are unlikely to survive learning events.
Brains are learning computers. Indeed, our brains appear to learn even while we are asleep (O’Neill et al. 2010; Warmsley & Stickgold 2010). It seems reasonable to suppose that computational roles inside the brain are not fixed but are constantly shifting, adapting to new information and trying out new strategies. In Schneider’s account, LOT symbol types disappear across these shifts. If LOT symbol types are so unstable and ephemeral, it is hard to see how generalisations involving them would be useful to science or philosophy.
It is worth emphasising that science needs LOT symbols that are stable across learning events. Recent work on LOT proposes that the brain’s learning algorithms perform probabilistic inference over LOT expressions (Piantadosi & Jacobs 2016; Piantadosi et al. 2016). In order for these algorithms to work, it is crucial that the identity of LOT expressions remains fixed across changes to their computational role so that the learner can consistently and rationally explore a space of hypotheses. Learning algorithms need to be defined over stable symbol types that do not themselves change during learning. Interestingly, this work tends to cite Feldman’s (2012) account of LOT symbol identity, which takes a semantic, broadly referential, approach to explaining what makes two (noisy, probabilistic) brain states of the same LOT symbol type. Schneider herself switches to a semantic method for individuating brain states when describing the computational principles shared between different humans – on her view, this is a non-computational way of individuating brain states.
4 Concepts and Frege cases
LOT says that concepts are LOT symbols and that the semantic value of a concept is purely referential. LOT therefore appears to have a problem with Frege cases: it cannot distinguish between co-referring concepts, at least not on purely semantic grounds. Fodor’s solution to this problem was to say that concepts should be individuated by both their semantic properties and their syntactic properties (Fodor 2008, Ch. 3). The concepts CICERO and TULLY have the same semantic content (referent), but they are distinct concepts because they involve two different LOT symbols.
Schneider endorses the same solution to Frege cases as Fodor, but she inserts her own theory of LOT symbol types. The result is a theory of concepts very different from what Fodor intended. Fodor called ‘pragmatism’ the claim that one’s concepts depend on one’s cognitive or behavioural capacities (including recognitional, classificatory, inferential capacities). According to a pragmatist, to have the concept DUCK is to be able to recognise ducks, classify ducks versus non-ducks, and perform inferences about ducks. Fodor thought pragmatism was ‘the defining catastrophe of analytic philosophy of language and philosophy of mind in the last half of the twentieth century’ (Fodor 2005 pp. 73–4). Schneider says that a LOT symbol’s identity, and hence a concept’s identity, depends on its total computational role, including its role in recognition, classification, and inference. Schneider’s theory of LOT symbols therefore entails Fodor’s hated pragmatism.
There is a delicious irony here, but should we accept Schneider’s theory of concepts? While not disputing her arguments, I would like to strike a note of caution. Schneider’s theory makes her concepts just as unstable and ephemeral as her LOT symbol types. She says that stability is provided by unvarying semantic referents. But an agent needs stable concepts, not just stable referents. In order for an agent’s inferences to be valid, the same concepts need to appear in her premises as in her conclusions. This won’t happen, or at least it is unlikely to happen, on Schneider’s view. Concepts tokened in a premise may not be around by the time the agent is ready to token her conclusion. If an agent were to learn just one new thing between tokening her premises and tokening her conclusion, her inference would likely be invalid as her concepts would likely have changed. The purpose of LOT is to mechanise thought. Concepts need to be stable for this; they need to hang around long enough for an agent to use them multiple times. Individuating concepts by their total computational role makes concepts too unstable. It does not allow LOT to achieve its goal.
5 Conclusion
This book throws into relief the difficulty, and importance, of the problem of individuating LOT symbols into symbol types. Contra Schneider, my instinct is to give a semantic solution to this problem. Unlike her, I’m not worried about LOT symbols presupposing semantic content. I think that reductive, naturalistic accounts of semantics already face more serious objections than a semantically inflected notion of symbols. There are also independent reasons to separate LOT from a reductive, naturalistic theory of content. LOT may be true and useful independently of the success or failure of such a theory. Indeed, many cognitive scientists who use LOT do not care much about the success of the project of naturalising semantics at all.
Schneider’s book advances the debate on LOT. She updates the theory by integrating considerations as diverse as neurocomputational models and neo-Russellianism about names. Her book wears its learning lightly, engaging the reader with simple examples and clearly motivated considerations. Whether or not you end up agreeing with all its claims, I would encourage you to buy and to read it.
Bibliography
Baars, B., & Franklin, S. (2003). ‘How conscious experience and working memory interact’, Trends in Cognitive Sciences, 7: 166–72.
Dehaene, S., & Changeux, J.-P. (2004). ‘Neural mechanisms for access to consciousness’. Gazzaniga M. (ed.) The cognitive neurosciences, III, pp. 1145–57. MIT Press: Cambridge, MA.
Feldman, J. (2012). ‘Symbolic representation of probabilistic worlds’, Cognition, 123: 61–83.
Fodor, J. A. (1975). The language of thought. Cambridge, MA: Harvard University Press.
——. (2000). The mind doesn’t work that way. Cambridge, MA: MIT Press.
——. (2005). Hume variations. Oxford: Oxford University Press.
——. (2008). LOT2: The language of thought revisited. Oxford: Oxford University Press.
Haugeland, J. (1985). Artificial intelligence: The very idea. Cambridge, MA: MIT Press.
Mnih, V., Kavukcuoglu, K., Silver, D., Rusu, A., Veness, J., Bellemare, M. G., Graves, A., et al. (2015). ‘Human-level control through deep reinforcement learning’, Nature, 518: 529–33.
O’Neill, J., Playdell-Bouverie, B., Dupret, D., & Csicsvari, J. (2010). ‘Play it again: Reactivation of waking experience and memory’, Trends in Neurosciences, 33: 220–9.
Patterson, D. A., & Hennessy, J. L. (2011). Computer organization and design: The hardware/software interface., 4th ed. Waltham, MA: Morgan Kaufmann.
Piantadosi, S. T., & Jacobs, R. A. (2016). ‘Four problems solved by the probabilistic language of thought’, Current Directions in Psychological Science, 25: 54–9.
Piantadosi, S. T., Tenenbaum, J. B., & Goodman, N. D. (2016). ‘The logical primitives of thought: Empirical foundations for compositional cognitive models’, Psychological Review, 123: 392–424.
Samuels, R. (2010). ‘Classical computationalism and the many problems of cognitive relevance’, Studies in History and Philosophy of Science, 41: 280–93.
Shanahan, M. (1997). Solving the frame problem. Cambridge, MA: Bradford Books, MIT Press.
Shanahan, M., & Baars, B. (2005). ‘Applying global workspace theory to the frame problem’, Cognition, 98: 157–76.
Warmsley, E. J., & Stickgold, R. (2010). ‘Dreaming and offline memory processing’, Current Biology, 20: R1010–3.